Abstract
Purpose. The current study sought to expand implications of physiological weathering through the application of latent profile analysis to stress biomarkers to address limitations of traditional allostatic load calculations.
Methods. Latent profile analysis was applied biomarkers used in traditional allostatic load metrics to identify physiological risk profiles in the 2007-20010 National Health and Nutritional and Examination Survey. Multinomial logistic regression was used to determine the probability of risk profiles by race/ethnicity, age, gender, and poverty income ratio (PIR). Mean allostatic load score was assessed across each risk profile.
Results. Latent profile analysis identified four distinct profiles labeled low risk, inflammatory risk, cardiovascular risk, and hypertension risk. Race, age, and gender significantly increased odds of exhibiting a risk profile. Compared to Whites, Hispanics had significant higher odds of inflammatory (OR=1.43, 95% CI [1.06-1.92]) and cardiovascular risk profiles (OR=1.63, 95% CI [1.09-2.43]) while Blacks had higher odds of inflammatory (OR=1.76 95% CI [1.25-2.47]), cardiovascular (OR=2.12, 95% CI, [1.39-3.27]) and hypertension risk profiles (OR= 1.78, 95% CI [1.21-2.59]). Females held significant greater odds of all risk categories except hypertension in which they held the lowest odds (OR= .19, 95% CI [.14-.25]). Mean allostatic load scores were highest in the inflammatory (M=3.99, SD=1.66) and cardiovascular risk profiles (M=4.4, SD=1.84).
Conclusions. Employing latent profile analysis may expand traditional allostatic load methodology by identifying physiological risk patterns among those who experience allostatic load early in life. This may be useful for examining how cultural specific interventions may reduce cardiovascular risk among those exhibiting physiological risk profiles.
License
This is an open access article distributed under the Creative Commons Attribution License which permits unrestricted use, distribution, and reproduction in any medium, provided the original work is properly cited.
Article Type: Research Article
EUR J ENV PUBLIC HLT, Volume 3, Issue 2, 2019, Article No: em0029
https://doi.org/10.29333/ejeph/5870
Publication date: 10 Aug 2019
Article Views: 3729
Article Downloads: 1915
Open Access HTML Content References How to cite this articleHTML Content
INTRODUCTION
Although rates of morbidity and mortality from cardiovascular disease have improved over the past decades, racial disparities in cardiovascular disease risk have persisted for ethnic and racial minorities (Berry et al., 2012; Go et al., 2014; Romero, Romero, Shlay, Ogden, and Dabelea, 2012). Research seeking to explain these disparities has largely focused on traditional risk factors such as obesity, hypertension, diet, and physical activity (Daviglus, Pirzada, and Talavera, 2014; Mathieu et al., 2012; Romero et al., 2012). However, a large body of literature has evidenced the psychological and social implications of race on cardiovascular risk (Jackson, McGibbon, and Waldron, 2013; Krieger et al., 2013; Sims et al., 2012). The weathering hypothesis posits that stress associated with racial inequities may cause health deterioration among African Americans as early as young adulthood, leading to racial disparities in health outcomes over the lifespan. Stress associated with economic, social, and structural inequities accelerates physiological erosion or “weathering” particularly among African American women (Geronimus, 2000; Geronimus and Thompson, 2004). Weathering is believed to create age patterns of morbidity and mortality among younger African Americans that are similar to older Whites thus creating differences in racial and age patterns in health outcomes of cardiovascular disease, infant mortality, death, and disability (Geronimus, 1996; Geronimus et al., 2010; Geronimus, Hicken, Keene, and Bound, 2006; Warren-Findlow, 2006).
Allostatic load is widely accepted as a quantitative metric used to study the consequences of weathering, thus giving researchers the ability to measure both the acute and long-term outcomes of the stress response. These efforts have been driven by literature linking social factors such as neighborhood quality, poverty, and occupation to physiological measures such as cortisol, blood pressure and levels of glycohemoglobin (Barrington et al., 2014; Schnorpfeil et al., 2003; Seeman, Singer, Wilkinson, and McEwen, 2001). Allostasis is the process that activates the sympathetic nervous system, hypothalamic-pituitary-adrenal axis (HPA) and the immune systems to adapt to external stressors. Prolonged allostasis results in allostatic load, a state of impaired ability to adapt to future stressors (Seplaki, Goldman, Weinstein, and Lin, 2004). The measurement of allostatic load is based on a set of biomarkers that represent the current state of each physiological system activated during stress exposure. Previous studies have employed an algorithm for computing allostatic load that includes measures of the HPA axis, cardiovascular system, metabolic processes and the immune system (Brody et al., 2013; Chyu and Upchurch, 2011; Geronimus et al., 2006; Seeman, Singer, Rowe, Horwitz, and McEwen, 1997). This algorithm computes allostatic load by demarcating clinical cut points to dichotomize continuous biological measurement into binary high-risk variables (0 = low, 1 = high). These binary variables (one for each biomarker) are then summed to yield an allostatic load score that determines the presence of allostatic load. Higher allostatic load, in general, has been associated with a decline in physical and cognitive functioning, cardiovascular disease incidence, and mortality (McEwen and Seeman, 1999). Moreover, evidence suggests that African Americans have a greater probability than Whites of a high allostatic load with racial differences growing more pronounced with increasing age (Geronimus et al., 2006).
Although this method of examining weathering has been foundational, there are several limitations to this approach (Geronimus et al., 2006; Seeman et al., 1997). First, clinical cut points used to create the binary variables may not capture the full extent of biological risk (Royston, Altman, and Sauerbrei, 2006). Second, categorizing continuous data results in loss of valuable information captured by variables used to calculate allostatic load (Altman, Lausen, Sauerbrei, and Schumacher, 1994; Cohen, 1983). This could be problematic when determining risk stratification for those who have elevated values but do not meet the clinical thresholds of risk. Third, it is improbable that each biomarker equally contributes to the calculation of an allostatic load score. A dichotomized composite score presents challenges when determining the extent to which a specific biomarker drives the overall allostatic load score.
This study proposes an expansion to this approach that explores whether latent profile analyses (LPA) using biomarkers commonly used to quantify allostatic load would be useful in capturing a more comprehensive extent of biological risk in a national sample of adults aged 18-65 years old (Masyn, 2013; Muthén, 2006). It was hypothesized that LPA would reveal risk profiles indicating various physiological risk patterns that could be useful for identifying predictors of sub-clinical cardiovascular disease risk.
In addition to addressing the limitations of the traditional methodology, the scope of the study was broadened to include a sample of Hispanics. Evidence of weathering has been consistent for African Americans but mixed among studies examining weathering among Hispanic populations (Collins, Rankin, and Hedstrom, 2012; Wildsmith, 2002). Therefore, the study sought to examine racial differences in memberships in physiological risk profile among Whites, African Americans, and Hispanics.
METHODS
Data from the 2007-2010 National Health and Nutritional Examination Survey (NHANES) provided the most recent national population level data with allostatic load metrics. NHANES collects data through both interviews (e.g., demographics) and examinations (e.g., physiological and laboratory) using a stratified multistage probability sample representing the United States population for civilian, noninstitutionalized individuals. The sample included males and females aged 18-65 years who self-identified as Black/African American, White, and Mexican/other Hispanic. Participants who were pregnant during examination were excluded from the study. We incorporated family poverty income ratio (PIR) calculated by dividing family income, specific to family size, by the Department of Health and Human Services’ poverty guidelines. NHANES oversampled individuals aged 60 and older, Blacks, and Hispanics, therefore, the Mobile Examination Center (MEC) weight variable was used to compensate for oversampling and those who were subject to examination procedures.
LPA was employed to develop physiological risk profiles. LPA is similar to cluster analysis in its use to identify subgroups within the sample based on a set of “indicator” variables (Masyn, 2013; Muthén, 2006). However, LPA employs maximum likelihood estimates of the probability of class membership and provides tools for decisions on which solutions to retain. LPA does not assume local independence, which fixes the within-class covariance among the input variables at zero. Relaxing this assumption of independence in LPA allows for flexibility in the structure of the classes concerning the means, variances and covariances of the indicator variables. This flexibility in class structure provides the opportunity to achieve better fitting models than more traditional approaches.
Generally, allostatic load metrics includes markers that measure primary mediators of stress (e.g., cortisol, epinephrine, norepinephrine) and outcomes associated with long-term stress exposure on the cardiovascular, metabolic, and immune systems. Due to biomarker availability within NHANES, indicator variables included 10 biomarkers; Body Mass Index (BMI), systolic blood pressure (SBP), diastolic blood pressure (DBP), pulse (PLS), C-reactive protein (CRP), high-density lipoprotein (HDL), total cholesterol (CHO), glycohemoglobin (GLY), albumin (ALB), and creatinine clearance (CREAT) (Juster, McEwen, and Lupien, 2010; McEwen and Seeman, 1999). Individuals with missing data for any indicator were excluded from the study.
Statistical Approach
Raw data for each biomarker was transformed into standardized Z- scores to account for different units of measurement across our ten biomarkers. Many of the biomarkers exhibited a highly positive skew. To present knowledge, there are no strong recommendations regarding the impact of skewness on LPA. Therefore, a systematic approach was adopted to trim the raw data. Three levels of trimming were established at the 99th percentile, 97th percentile, and the 95th percentile. Using the raw data and the three levels of trimming, a series of LPA models were estimated ranging from 2 – 6 classes. Models with untrimmed data often did not converge, creating a rationale for utilizing trimmed data. Trimming analysis revealed trimming at the 99th percentile resulted in the best fit.
Using Mplus version 7, (Muthén and Muthén, 1998) we first examined a series of LPA models by increasing the number of classes (from 1 to 6). We examined changes across the number of classes with respect to the following fit indices; Akaike Information Criterion (AIC), Bayesian Information Criterion (BIC), and the Sample Size Adjusted Bayesian Information Criterion (SS-BIC) (Geiser, 2012). Second, we employed the Lo-Mendell-Rubin (LMR) test to determine the appropriateness of K versus K-1 number of classes. We used entropy statistics to determine classification accuracy. Entropy values range from 0-1 where values closer to 1 represent a higher classification accuracy (Geiser, 2012). We conducted multinomial logistic regression analysis using Mplus to determine odds of class membership (Asparouhov and Muthén, 2014). Predictor variables included race/ethnicity (Black, White, Hispanic), sex, age and PIR. Preliminary analysis revealed that odds of class membership increased for every one year increase in age across each risk profile. Therefore, it was more meaningful to investigate age as the following categories: 18-24; 25-34; 35-44; 45-54; and 55-64 years.
For each risk profile identified, a mean allostatic load score was calculated to determine its overall physiological burden (Juster et al., 2010). A binary coding method using clinical cut-points was used to assign participants a point for a reading beyond a clinical high-risk threshold. Values for clinical cut points were determined based on existing allostatic load literature (Geronimus et al., 2006; Juster et al., 2010). The cut points were as followed: SBP, 140 mm Hg; DBP, 90 mm Hg; PLS, 90 bpm, HDL, less than 50 mg/dL; BMI, 30; GLY, 6.4%; CRP, .40 mg/dL; ALB, less 4 g/dL; CHO, 240 mg/dL; CREAT, less than 65 mg/dL (estimated using the Cockcroft-Gault equation) (Cockcroft and Gault, 1976). Participants who reported taking medications for high blood pressure, cholesterol or diabetes were also assigned a point. Points were then summed to obtain a total allostatic load score for each participant.
RESULTS
Latent Profile Model
Using a total sample size of 4047, series of models were used to determine the best model fit indicated by the lowest value of fit indices (e.g., AIC, BIC, ssBIC) (Table 1). Although the Lo-Mendel Rubin test was significant in favor of a 5-class model vs. 4-class (p= .0076) there were relatively minor differences in the fit indices between the 4- vs 5-class solutions. Further, two profiles yielded patterns indicating inflammatory risk in the 5-class solution. Therefore, this information was used collectively to determine that the 4-class model presented the most coherent conceptual interpretability. The standardized means for each biomarker in the five profiles are depicted in Figure 1.
Table 1. Fit Indices for General Latent Profile Analyses
|
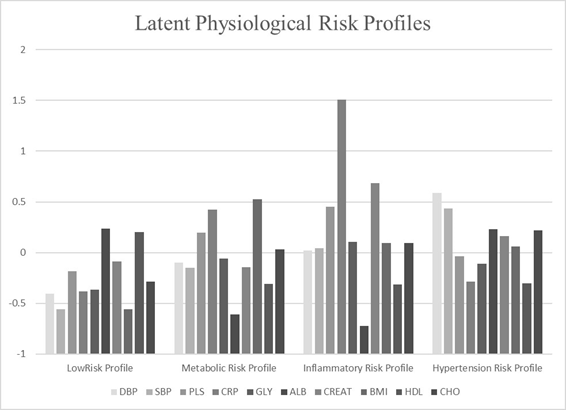
Latent profile classes
The first profile (n= 1786, 41.14%) yielded the largest number of participants (Table 2). This profile was categorized by higher levels of albumin (z= .236) and HDL (z= .202) which have been associated with better health outcomes (Horwich, Kalantar-Zadeh, MacLellan, and Fonarow, 2008). Therefore, this profile was classified as the “low risk profile”. This profile exhibited the lowest mean age (36 years, SD=21.30) and had the lowest mean allostatic load score (M= 1.10, SD=1.09). Approximately 56% of the participants in this profile were female. The second profile (n=611 15.1%) was labeled the “cardiovascular risk profile” due the higher pulse rates (z=.197), BMI (z=.528), and C-reactive protein (z=.424) in this profile. The mean allostatic load score in the cardiovascular risk profile was 3.99 (SD=1.66) while the mean age was 43.60 (SD=13.57). The third profile comprised the smallest number of participants (n=259, 6.3%) and labeled the “inflammatory risk profile” due to the considerably elevated levels of C-reactive protein (z=1.506). This profile had the highest mean allostatic load score (M= 4.4, SD=1.84) and the second highest mean age (M=44.41, SD=13.55). The majority of participants in this profile were females (70.7%). The last profile exhibited high levels of systolic (z= 0.588) and diastolic blood pressure (z=0.437) thus we labeled this profile “hypertension risk”. The mean allostatic load score for this profile (M= 2.72, SD=1.87) and this profile revealed the highest mean age (M=47.21, SD=12.10). The profile had the highest percentage of males (66.7%).
Table 2. Mean comparisons between Latent profiles for Mean Allostatic Load (AL), Latent Profile Indicators and External Variables
|
Multinomial logistic regression was conducted using the low risk profile as the referent group (Table 3). Compared to Whites, Hispanics participants had significantly greater odds of an inflammatory (OR= 1.43, 95% CI [1.06-1.92]) and cardiovascular risk profiles (OR=1.63, 95% CI [1.09-2.43]) while Black participants held significantly greater odds of inflammatory (OR= 1.75, 95% CI [1.25-2.47]), cardiovascular (OR= 2.12, 95% CI [1.39-3.27]), and hypertension (OR=1.76, 95% CI [1.21-2.59]) risk profiles. In contrast to males, females had a higher odds of a cardiovascular risk profile (OR=1.59, 95% CI [1.022-2.47]) but significantly lower in the hypertension profile (OR=.19, 95% CI [.14-.25]). No significant relationship was observed between PIR and risk profiles. Using age 18-24 as referent category, the odds of an inflammatory risk profile were significantly higher for ages 25-34 years (OR=1.96, 95% CI [1.19-3.23], 35-44 years (OR=2.48, 95% CI [1.49-4.14]), 45-54 years (OR=3.68, 95% CI [2.12-6.37]), and 55-65 years (OR=5.92, 95% CI [3.24-10.78]). Significant increases in the odds of a cardiovascular risk profile were only observed among those 45-54 years (OR=5.33, 95% CI [2.70-10.52]) and 55-65 years (OR=6.39, 95% CI [2.98-13.67]). The largest age-related odds were seen in the hypertension risk profile where the odds significantly increased across ages 25-34 years (OR=6.78, 95% CI [2.77-16.6]), 35-44 years (OR=18.08, 95% CI [7.48-43.68], 45-54 years (OR=34.09, 95% CI [13.94-83.33]) and 55-65 years (OR= 75.79 95% CI [29.88-192.29]).
Table 3. Odds Ratio for Latent Profile Classes with Race, Sex, Age, and PIR as Predictors using the Low Risk Profile as Referent
|
Discussion/Conclusion
Applying latent profile analysis to continuous level variables revealed four distinct physiological risk profile groups. The weathering hypothesis assumes that stress accelerates physiological erosion over time. Though the impact of stress was unable to be assessed in this study, each profile differed by not only mean allostatic load score but race/ethnicity, gender, and age category. In the inflammatory risk profile, higher levels of C- reactive protein values were associated with lower levels of albumin. This is consistent with the literature indicating a systematic inflammatory state (Kuller et al., 1991; Menon et al., 2005). This profile had the highest mean allostatic load score indicating the presence of physiological weathering among members who exhibited this physiological risk profile. Hispanic and Black participants held greater odds of an inflammatory risk profile. This profile may portray a physiological patterning of weathering that increases the risk of allostatic load among Black and Hispanic populations. In the context of the weathering hypothesis, social stressors such as race and gender discrimination are often reported by Black populations and have been associated with elevated levels of C-reactive protein (Lewis, Aiello, Leurgans, Kelly, and Barnes, 2010). This may warrant further study of the relationship between subjective stress experiences among individuals who fit this physiological profile, particularly Black and Hispanic females.
Increased C-reactive protein, BMI, and pulse rates characterized the cardiovascular risk profile. Previous studies have suggested pulse rate and C-reactive protein are higher among individuals with higher BMI (Martins, Tareen, Pan, and Norris, 2003; Visser, Bouter, McQuillan, Wener, and Harris, 1999). These risk factors together have been associated with increased cardiovascular disease risk (Diaz, Bourassa, Guertin, and Tardif, 2005; Menon et al., 2005). This may be more evident among those who are normotensive as suggested by the mean systolic and diastolic pressure (120/70 mmHg) in this profile (Martins et al., 2003). This profile exhibited a similar mean allostatic load score as the inflammatory risk profile suggesting those in the cardiovascular risk profile could also be experiencing some form of physiological weathering. This is particularly evident for Blacks and Hispanics participants who also held greater odds of exhibiting this profile than Whites. However, these significant differences in the odds were only observed for those 45-54 and 55-65 years. Therefore, the potential impact of age-related physiological deterioration cannot be discounted when examining drivers of allostatic load scores in this profile.
The hypertension risk profile was characterized by elevated mean systolic and diastolic blood pressure. It was not unexpected to observe large odd ratios that increased across each age category. These large odds ratios may be attributed to the low percentage of those 18-24 years (4.9%) in this profile. Participants 45-54 years (27.8%) and 55-65 (32.9%) accounted for the majority of individuals who fit this profile. Given the age distribution and mean systolic and diastolic blood pressure in the profile, it was surprising to observe a mean allostatic load score of 2.72. Moreover, individuals who identified as Black and male had greater odds of being in this profile suggesting that Black men may experience hypertension risk without exhibiting allostatic load. These findings prompt further inquiry to understand the relationship between hypertension risk and allostatic load, particularly for Black men.
The present study held several limitations. This is a cross-sectional study that explored the relationship between physiological risk profile groups and the likelihood of physiological risk profile across population subgroups. Therefore, the relationship between a particular risk profile and specific cardiovascular events were unable to be assessed. Further, the most comprehensive examination of allostatic load encompasses both markers of primary mediators of stress and secondary outcomes on the immune, cardiovascular, and metabolic systems (Juster et al., 2010; McEwen and Seeman, 1999). Our study only included secondary outcomes of stress.
In conclusion, the focus of the study was to explore our ability to expand the conceptualization of allostatic load by applying latent profile analysis to address limitations in traditional allostatic load calculations. This line of inquiry is in the initial stages. Yet, the manifestation of these four profiles in a national sample may illustrate different patterns of physiological weathering among qualitatively distinct population groups. This is significant as risk profiles were categorized by self-identification as African American or Hispanic, sex, and age stratification. Thus, these profiles may display potential physiological patterns of risk that could elucidate various pathways in which racial inequities lead to early aged allostatic load. This may be useful in identifying individuals who have a sub-clinical cardiovascular disease risk. Moreover, researchers can continue to examine how cultural and age-specific coping efforts with other resources such as social support can reduce the biological risk for African American and Hispanics exhibiting specific physiological risk profiles.
References
- Altman, D. G., Lausen, B., Sauerbrei, W. and Schumacher, M. (1994). Dangers of using “optimal” cutpoints in the evaluation of prognostic factors. Journal of the National Cancer Institute, 86(11), 829–835. https://doi.org/10.1093/jnci/86.11.829
- Asparouhov, T. and Muthén, B. (2014). Auxiliary variables in mixture modeling: Three-step approaches using Mplus. Structural Equation Modeling: A Multidisciplinary Journal, 21(3), 329–341. https://doi.org/10.1080/10705511.2014.915181
- Barrington, W. E., Stafford, M., Hamer, M., Beresford, S. A., Koepsell, T. and Steptoe, A. (2014). Neighborhood socioeconomic deprivation, perceived neighborhood factors, and cortisol responses to induced stress among healthy adults. Health & Place, 27, 120–126. https://doi.org/10.1016/j.healthplace.2014.02.001
- Berry, J. D., Dyer, A., Cai, X., Garside, D. B., Ning, H., Thomas, A., et al. (2012). Lifetime risks of cardiovascular disease. New England Journal of Medicine, 366(4), 321–329. https://doi.org/10.1056/NEJMoa1012848
- Brody, G. H., Yu, T., Chen, Y., Kogan, S. M., Evans, G. W., Beach, S. R. H., et al. (2013). Cumulative Socioeconomic Status Risk, Allostatic Load, and Adjustment: A Prospective Latent Profile Analysis With Contextual and Genetic Protective Factors. Developmental Psychology, 49(5), 913–927. https://doi.org/10.1037/a0028847
- Chyu, L. and Upchurch, D. M. (2011). Racial and ethnic patterns of allostatic load among adult women in the United States: findings from the National Health and Nutrition Examination Survey 1999–2004. Journal of Women’s Health, 20(4), 575–583. https://doi.org/10.1089/jwh.2010.2170
- Cockcroft, D. W. and Gault, H. (1976). Prediction of creatinine clearance from serum creatinine. Nephron, 16(1), 31–41. https://doi.org/10.1159/000180580
- Cohen, J. (1983). The cost of dichotomization. Applied psychological measurement, 7(3), 249-253. https://doi.org/10.1177/014662168300700301
- Collins, J. W., Rankin, K. M. and Hedstrom, A. B. (2012). Exploring weathering: the Relation of age to low birth weight among first generation and established United States-Born Mexican–American Women. Maternal and Child Health Journal, 16(5), 967–972. https://doi.org/10.1007/s10995-011-0827-4
- Daviglus, M. L., Pirzada, A. and Talavera, G. A. (2014). Cardiovascular disease risk factors in the Hispanic/Latino population: lessons from the Hispanic Community Health Study/Study of Latinos (HCHS/SOL). Progress in Cardiovascular Diseases, 57(3), 230–236. https://doi.org/10.1016/j.pcad.2014.07.006
- Diaz, A., Bourassa, M. G., Guertin, M.-C. and Tardif, J.-C. (2005). Long-term prognostic value of resting heart rate in patients with suspected or proven coronary artery disease. European Heart Journal, 26(10), 967–974. https://doi.org/10.1093/eurheartj/ehi190
- Geiser, C. (2012). Data analysis with Mplus. Guilford Press. https://doi.org/10.1007/978-3-531-93192-0
- Geronimus, A. T. (1996). Black/white differences in the relationship of maternal age to birthweight: a population-based test of the weathering hypothesis. Social Science & Medicine, 42(4), 589–597. https://doi.org/10.1016/0277-9536(95)00159-X
- Geronimus, A. T. (2000). Understanding and eliminating racial inequalities in women’s health in the United States: the role of the weathering conceptual framework. Journal of the American Medical Women’s Association (1972), 56(4), 133–6.
- Geronimus, A. T., Hicken, M., Keene, D. and Bound, J. (2006). “Weathering” and age patterns of allostatic load scores among blacks and whites in the United States. American Journal of Public Health, 96(5), 826–833. https://doi.org/10.2105/AJPH.2004.060749
- Geronimus, A. T., Hicken, M. T., Pearson, J. A., Seashols, S. J., Brown, K. L. and Cruz, T. D. (2010). Do US black women experience stress-related accelerated biological aging? Human Nature, 21(1), 19–38. https://doi.org/10.1007/s12110-010-9078-0
- Geronimus, A. T. and Thompson, J. P. (2004). To denigrate, ignore, or disrupt: racial inequality in health and the impact of a policy-induced breakdown of African American communities. Du Bois Review, 1(02), 247–279. https://doi.org/10.1017/S1742058X04042031
- Go, A. S., Mozaffarian, D., Roger, V. L., Benjamin, E. J., Berry, J. D., Blaha, M. J., et al. (2013). Heart disease and stroke statistics—2014 update: a report from the American Heart Association. Circulation, 01-cir. https://doi.org/10.1161/CIR.0b013e31828124ad
- Horwich, T. B., Kalantar-Zadeh, K., MacLellan, R. W. and Fonarow, G. C. (2008). Albumin levels predict survival in patients with systolic heart failure. American Heart Journal, 155(5), 883–889. https://doi.org/10.1016/j.ahj.2007.11.043
- Jackson, J., McGibbon, E. and Waldron, I. (2013). Racism and cardiovascular disease: implications for nursing. Canadian Journal of Cardiovascular Nursing, 23(4).
- Juster, R.-P., McEwen, B. S. and Lupien, S. J. (2010). Allostatic load biomarkers of chronic stress and impact on health and cognition. Neuroscience & Biobehavioral Reviews, 35(1), 2–16. https://doi.org/10.1016/j.neubiorev.2009.10.002
- Krieger, N., Waterman, P. D., Kosheleva, A., Chen, J. T., Smith, K. W., Carney, D. R., et al. (2013). Racial discrimination & cardiovascular disease risk: my body my story study of 1005 US-born black and white community health center participants (US). PloS One, 8(10), e77174. https://doi.org/10.1371/journal.pone.0077174
- Kuller, L. H., Eichner, J. E., Orchard, T. J., Grandits, G. A., McCallum, L., Tracy, R. P., et al. (1991). The relation between serum albumin levels and risk of coronary heart disease in the Multiple Risk Factor Intervention Trial. American Journal of Epidemiology, 134(11), 1266–1277. https://doi.org/10.1093/oxfordjournals.aje.a116030
- Lewis, T. T., Aiello, A. E., Leurgans, S., Kelly, J. and Barnes, L. L. (2010). Self-reported experiences of everyday discrimination are associated with elevated C-reactive protein levels in older African-American adults. Brain, Behavior, and Immunity, 24(3), 438–443. https://doi.org/10.1016/j.bbi.2009.11.011
- Martins, D., Tareen, N., Pan, D. and Norris, K. (2003). The relationship between body mass index, blood pressure and pulse rate among normotensive and hypertensive participants in the third National Health and Nutrition Examination Survey (NHANES). Cellular and Molecular Biology, 49(8), 1305–1309.
- Masyn, K. (2013). Latent class analysis and finite mixture modeling. The Oxford Handbook of Quantitative Methods in Psychology, 2, 551–611. https://doi.org/10.1093/oxfordhb/9780199934898.013.0025
- Mathieu, R. A., Powell-Wiley, T. M., Ayers, C. R., McGuire, D. K., Khera, A., Das, S. R. and Lakoski, S. G. (2012). Physical activity participation, health perceptions, and cardiovascular disease mortality in a multiethnic population: The Dallas Heart Study. American Heart Journal, 163(6), 1037–1040. https://doi.org/10.1016/j.ahj.2012.03.005
- McEwen, B. S. and Seeman, T. (1999). Protective and damaging effects of mediators of stress: elaborating and testing the concepts of allostasis and allostatic load. Annals of the New York Academy of Sciences, 896(1), 30–47. https://doi.org/10.1111/j.1749-6632.1999.tb08103.x
- Menon, V., Greene, T. O. M., Wang, X., Pereira, A. A., Marcovina, S. M., Beck, G. J., … Sarnak, M. J. (2005). C-reactive protein and albumin as predictors of all-cause and cardiovascular mortality in chronic kidney disease. Kidney International, 68(2), 766–772. https://doi.org/10.1111/j.1523-1755.2005.00455.x
- Muthén, B. (2006). The potential of growth mixture modeling. Infant and Child Development, 15(6), 623–625. https://doi.org/10.1002/icd.482
- Muthén, L. K. and Muthén, B. O. (1998). MPLUS User’s Guide, Seventh edition.
- Romero, C. X., Romero, T. E., Shlay, J. C., Ogden, L. G. and Dabelea, D. (2012). Changing trends in the prevalence and disparities of obesity and other cardiovascular disease risk factors in three racial/ethnic groups of USA adults. Advances in Preventive Medicine, 2012. https://doi.org/10.1155/2012/172423
- Royston, P., Altman, D. G. and Sauerbrei, W. (2006). Dichotomizing continuous predictors in multiple regression: a bad idea. Stat Med, 25(1), 127–141. https://doi.org/10.1002/sim.2331
- Schnorpfeil, P., Noll, A., Schulze, R., Ehlert, U., Frey, K. and Fischer, J. E. (2003). Allostatic load and work conditions. Social Science & Medicine, 57(4), 647–656. https://doi.org/10.1016/S0277-9536(02)00407-0
- Seeman, T. E., Singer, B. H., Rowe, J. W., Horwitz, R. I. and McEwen, B. S. (1997). Price of adaptation—allostatic load and its health consequences: MacArthur studies of successful aging. Archives of Internal Medicine, 157(19), 2259–2268. https://doi.org/10.1001/archinte.1997.00440400111013
- Seeman, T. E., Singer, B., Wilkinson, C. W. and McEwen, B. (2001). Gender differences in age-related changes in HPA axis reactivity. Psychoneuroendocrinology, 26(3), 225–240. https://doi.org/10.1016/S0306-4530(00)00043-3
- Seplaki, C. L., Goldman, N., Weinstein, M. and Lin, Y.-H. (2004). How are biomarkers related to physical and mental well-being? The Journals of Gerontology Series A: Biological Sciences and Medical Sciences, 59(3), B201–B217. https://doi.org/10.1093/gerona/59.3.B201
- Sims, M., Diez-Roux, A. V., Dudley, A., Gebreab, S., Wyatt, S. B., Bruce, M. A., et al. (2012). Perceived discrimination and hypertension among African Americans in the Jackson Heart Study. American Journal of Public Health, 102(S2), S258–S265. https://doi.org/10.2105/AJPH.2011.300523
- Visser, M., Bouter, L. M., McQuillan, G. M., Wener, M. H. and Harris, T. B. (1999). Elevated C-reactive protein levels in overweight and obese adults. Jama, 282(22), 2131–2135. https://doi.org/10.1001/jama.282.22.2131
- Warren-Findlow, J. (2006). Weathering: Stress and heart disease in African American women living in Chicago. Qualitative Health Research, 16(2), 221–237. https://doi.org/10.1177/1049732305278651
- Wildsmith, E. M. (2002). Testing the weathering hypothesis among Mexican-origin women. Ethnicity and Disease, 12(4), 470–479.
How to cite this article
Vancouver
Johnson AJ, Dudley WN, Wideman L, Schulz M. Physiological Risk Profiles and Allostatic Load: Using Latent Profile Analysis to Examine Socioeconomic Differences in Physiological Patterns of Risk. EUR J ENV PUBLIC HLT. 2019;3(2):em0029. https://doi.org/10.29333/ejeph/5870
APA
Johnson, A. J., Dudley, W. N., Wideman, L., & Schulz, M. (2019). Physiological Risk Profiles and Allostatic Load: Using Latent Profile Analysis to Examine Socioeconomic Differences in Physiological Patterns of Risk. European Journal of Environment and Public Health, 3(2), em0029. https://doi.org/10.29333/ejeph/5870
AMA
Johnson AJ, Dudley WN, Wideman L, Schulz M. Physiological Risk Profiles and Allostatic Load: Using Latent Profile Analysis to Examine Socioeconomic Differences in Physiological Patterns of Risk. EUR J ENV PUBLIC HLT. 2019;3(2), em0029. https://doi.org/10.29333/ejeph/5870
Chicago
Johnson, Amber J., William N. Dudley, Laurie Wideman, and Mark Schulz. "Physiological Risk Profiles and Allostatic Load: Using Latent Profile Analysis to Examine Socioeconomic Differences in Physiological Patterns of Risk". European Journal of Environment and Public Health 2019 3 no. 2 (2019): em0029. https://doi.org/10.29333/ejeph/5870
Harvard
Johnson, A. J., Dudley, W. N., Wideman, L., and Schulz, M. (2019). Physiological Risk Profiles and Allostatic Load: Using Latent Profile Analysis to Examine Socioeconomic Differences in Physiological Patterns of Risk. European Journal of Environment and Public Health, 3(2), em0029. https://doi.org/10.29333/ejeph/5870
MLA
Johnson, Amber J. et al. "Physiological Risk Profiles and Allostatic Load: Using Latent Profile Analysis to Examine Socioeconomic Differences in Physiological Patterns of Risk". European Journal of Environment and Public Health, vol. 3, no. 2, 2019, em0029. https://doi.org/10.29333/ejeph/5870